일 | 월 | 화 | 수 | 목 | 금 | 토 |
---|---|---|---|---|---|---|
1 | ||||||
2 | 3 | 4 | 5 | 6 | 7 | 8 |
9 | 10 | 11 | 12 | 13 | 14 | 15 |
16 | 17 | 18 | 19 | 20 | 21 | 22 |
23 | 24 | 25 | 26 | 27 | 28 |
- 텍스트 분류
- 차원축소
- 텐서플로2와 머신러닝으로 시작하는 자연어처리
- 구글 BERT의 정석
- 가설검정
- 모두의 딥러닝
- numpy
- 회귀분석
- 밑바닥부터 시작하는 딥러닝
- 군집화
- 코사인 유사도
- 오래간만에 글쓰네
- student t분포
- 다층 퍼셉트론
- rnn
- 밑바닥부터 시작하는 딥러닝2
- Pandas
- 감성분석
- 머신러닝
- Django
- word2vec
- 은준아 화이팅
- 파이썬 pandas
- 기초통계
- 히스토그램
- 결정계수
- F분포
- 자연어 처리
- 최소자승법
- 기술통계학
- Today
- Total
목록Python/시각화 (4)
데이터 한 그릇
통계청 신생아수 데이터 matplotlib 시각화 기술통계 시각화 작업을 해봄. => 기본적인 전처리와 Seaborn의 다양한 그래프를 사용해봤다. df['variable'].str.split('.', expand = True) #의 return 은 DataFrame임. df['variable'].str.split('.', expand = True)[index] #는 return 이 Series임. 전처리 중에 df['variable'].str.split('.', expand = True) 를 사용하여 전처리 하는 쉬운 방법 알아냄 (이 함수는 split을 모든 행에 적용시켜준다.) for i in df['variable']: df['연도'] = i.split('.')[0] df['월'] = i.spli..
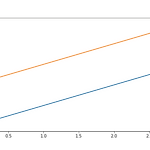
기본 matplotlib 함수들 plt.figure(figsize=()) plt.axes() plt.plot() plt.title() plt.grid() plt.xlabel() plt.ylabel() plt.xticks() plt.yticks() plt.xlim() plt.style.use() plt.subplot(x,y,z) fig, ax = plt.subplots(x,y) => fig, (axes1,axes2) =plt.subplots(x,y) import numpy as np import pandas as pd import matplotlib.pyplot as plt import seaborn as sns matplotlib 패키지와 seaborn 패키지 중에 matplotlib을 살펴볼 예정 pl..